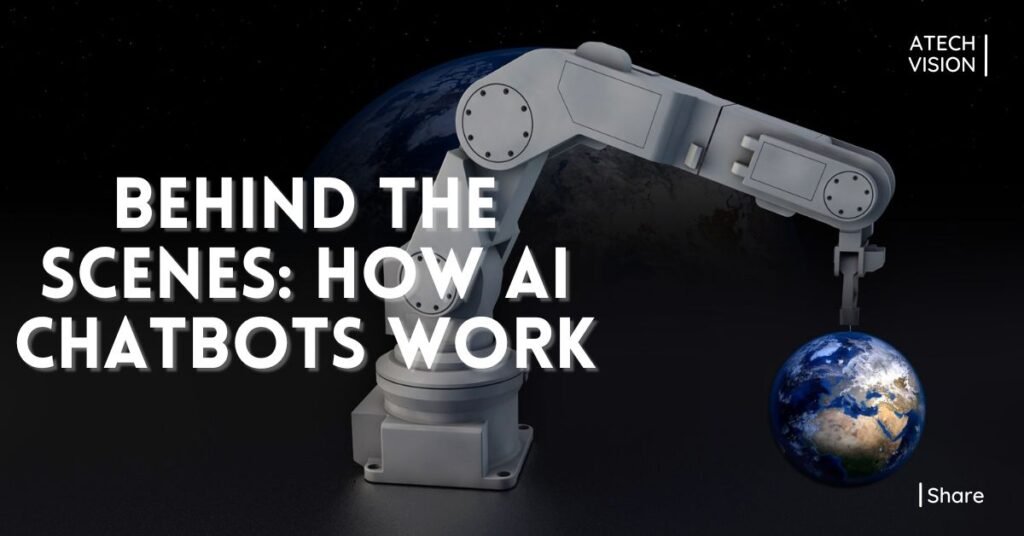
Artificial intelligence (AI) chatbots are revolutionizing how we interact with technology, powering virtual assistants, customer support systems, and more. These bots have transitioned from simple rule-based systems to complex, conversational agents capable of understanding and generating natural language. In this detailed exploration, we’ll unravel the components, technologies, and mechanisms that make AI chatbots work.
1. What Are AI Chatbots?
An AI chatbot is a software application that uses artificial intelligence to simulate conversations with users. Unlike traditional bots that rely on predefined responses, AI chatbots leverage advanced technologies like machine learning (ML) and natural language processing (NLP) to understand context, intent, and even emotion.
Types of Chatbots
• Rule-Based Chatbots: Operate using predefined rules and responses.
• AI-Powered Chatbots: Use NLP, ML, and other AI techniques to improve interaction and provide more dynamic responses.
• Hybrid Chatbots: Combine rule-based logic with AI capabilities for enhanced functionality.
2. Key Components of AI Chatbots
Natural Language Processing (NLP)
NLP is the cornerstone of chatbot functionality, enabling machines to understand and generate human-like text. It has two primary components:
1. Natural Language Understanding (NLU): Deciphers the meaning, intent, and context of user inputs.
2. Natural Language Generation (NLG): Produces coherent and contextually relevant responses.
Machine Learning (ML) Models
ML allows chatbots to learn from data and improve over time. Popular techniques include:
• Supervised Learning: Training models with labeled data to predict outcomes.
• Reinforcement Learning: Improving decision-making by rewarding desirable outcomes.
• Unsupervised Learning: Finding patterns in unlabeled data for better understanding.
Dialogue Management
This component determines the flow of conversation. It uses:
• Intent Recognition: Identifies what the user wants to achieve.
• Context Management: Keeps track of the conversation’s history to maintain continuity.
Knowledge Base and Databases
AI chatbots rely on structured and unstructured data sources to provide accurate information. Examples include FAQs, product databases, and knowledge graphs.
3. Core Technologies Behind AI Chatbots
1. Neural Networks
AI chatbots use neural networks to process text and generate responses. Popular architectures include:
• Recurrent Neural Networks (RNNs): Ideal for sequential data.
• Transformer Models: Advanced architectures like GPT (Generative Pre-trained Transformers) and BERT (Bidirectional Encoder Representations from Transformers) have significantly improved conversational AI.
2. Pre-Trained Language Models
State-of-the-art models such as OpenAI’s GPT and Google’s LaMDA (Language Model for Dialogue Applications) have transformed chatbot development. These models are trained on vast datasets, enabling them to understand nuanced language.
3. Speech-to-Text and Text-to-Speech
Voice-enabled chatbots incorporate these technologies to convert spoken language into text and vice versa.
4. API Integration
Chatbots connect to external systems (e.g., CRMs, databases) using APIs to fetch real-time data and perform tasks like booking, ordering, or retrieving information.
4. How Do Chatbots Learn?
AI chatbots improve through training processes that involve data preparation, model selection, and evaluation.
Training Data
Chatbots are trained on diverse datasets that include:
• Conversations
• User Feedback
• Domain-specific texts (e.g., medical or legal documents)
Continuous Learning
• Retraining Models: Developers update the bot’s training data periodically.
• User Feedback: Responses are fine-tuned based on user interaction data.
5. The Workflow of AI Chatbots
1. Input Recognition:
The user inputs a query via text or voice.
2. Preprocessing:
Text is cleaned, tokenized, and normalized for processing.
3. Intent Detection and Entity Recognition:
NLP algorithms identify the user’s intent (e.g., “book a flight”) and extract key entities (e.g., destination, date).
4. Response Generation:
Based on the intent and context, the chatbot formulates a response using NLG or retrieves one from its knowledge base.
5. Output Delivery:
The bot presents the response, either as text or voice.
6. Advanced Features and Capabilities
1. Context Awareness
Modern chatbots remember previous interactions to provide more meaningful responses. For example, a chatbot assisting with flight bookings can recall the destination discussed earlier.
2. Sentiment Analysis
By analyzing the tone of the user’s input, chatbots can detect emotions such as frustration or happiness, adjusting their responses accordingly.
3. Personalization
AI chatbots utilize user data to tailor responses. For instance, they might recommend products based on past purchases.
7. Real-world Applications
• Customer Support: Automating FAQs, complaint resolution, and troubleshooting.
• Healthcare: Scheduling appointments and providing symptom-checking tools.
• E-Commerce: Assisting with product searches, recommendations, and order tracking.
• Education: Interactive learning and language practice.
8. Ethical and Technical Challenges
Ethical Concerns
• Bias in AI: Training data may inadvertently introduce bias.
• Privacy Risks: Ensuring data security is critical for maintaining trust.
Technical Limitations
• Understanding Nuance: Chatbots may struggle with sarcasm, idioms, or ambiguous queries.
• Language Barriers: Multilingual capabilities are still evolving.
9. The Future of AI Chatbots
AI chatbots are expected to become more human-like and intuitive, integrating seamlessly into daily life. Future advancements may include:
• Emotionally Intelligent Bots: Capable of empathizing with users.
• Enhanced Multimodal Interaction: Combining text, voice, and visual inputs.
• Self-Learning Bots: Continuously improving without manual intervention.
References for Further Reading
• How AI Chatbots Work – Insights from Analytics Insight